What is the circular economy?
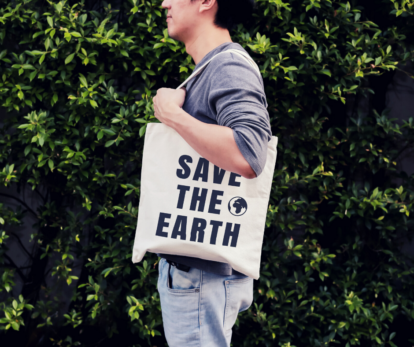
Artificial intelligence (AI) is revolutionising the way we live and work. From voice assistants and grammar checkers to Netflix recommendations and ChatGPT, incarnations of this rapidly evolving field are all around us and, indeed, so integral to many lives in the (wealthier parts of the) modern world that it’s already hard to imagine what we did without it.
People are already looking to the future of this field. There’s the promise of self-driving cars by 2035; smart cities where waste management and urban planning are AI-controlled; healthcare innovations and research methods that may finally replace testing on our animal kin; chatbots that are more human and nuanced in their responses; AI in agriculture – the list goes on and on.
But what do we mean by AI? Is it a new field or a long time in development? What are the most common types of AI, and why are they important? How might they impact people, especially those of us in the vegan community?
Let’s take a look…
Simply put, Artificial Intelligence (AI) is a field that “leverages computers and machines to mimic the problem-solving and decision-making capabilities of the human mind” (Source: IBM).
Before we look at the different types of AI, we thought we’d take you on a quick tour of the history of this world-changing technology.
The birth of Artificial Intelligence (1950s)
The origins of AI can be traced back to World War 2 and the work of cryptographers such as Alan Turing and Gordon Welchman to crack German military codes using early computing machines. The algorithms and machines developed for this purpose laid the groundwork for future machine-learning algorithms and even natural language processing, both of which are at the core of AI.
In 1956, computer scientist and cognitive scientist John McCarthy organised the Dartmouth Summer Research Project on Artificial Intelligence, which marked the birth of AI as a distinct field.
Participants discussed the potential for machines to exhibit intelligence and proposed that “every aspect of learning or any other feature of intelligence can in principle be so precisely described that a machine can be made to simulate it”. It was at this conference that McCarthy first coined the phrase “artificial intelligence”.
The AI early years (1950s to 1960s)
During this time period, researchers focused on developing programs capable of performing logical reasoning and solving mathematical problems. Significant work was done on early AI programs such as the Logic Theorist and the General Problem Solver.
The Logic Theorist was a program written in 1956 by Allen Newell, Herbert A Simon, and Cliff Shaw. It was designed to perform what’s known as “automated reasoning” and successfully proved 38 of the first 52 theorems in the Principia Mathematica. It is even said to have “found new and more elegant proofs” of these theorems.
A year later, Newell, Simon and Shaw created the General Problem Solver (GPS, but not the GPS we know today!), which is the first computer program to separate the knowledge of problems (i.e., the rules that it should apply to data) from the strategy of how to solve a problem.
Developing expert AI systems (1960s to 1980s)
Expert systems emerged as a popular AI approach during this period. These systems aimed to capture and replicate the knowledge and expertise of human specialists in specific domains. Examples include Dendral, which analysed chemical compounds, and MYCIN, used for diagnosing infectious diseases.
These systems are divided into two different subsystems – the first is the knowledge base, which represents known facts and rules, and the second is the inference engine, which takes known facts and uses them to deduce new facts.
The AI Winter (1970s-1980s)
Due to unmet expectations and overhyped promises, AI experienced a period known as the “AI Winter.” Funding and interest declined as early AI technologies failed to deliver on their promises, leading to a slowdown in research and development.
While some talked about the AI winter as though it was the end of the AI story, many researchers and computer scientists continued to explore how AI technology could be developed and implemented.
Machine Learning and Neural Networks (1980s-1990s)
Researchers shifted their focus to machine learning techniques, such as neural networks, which simulate the behaviour of the human brain. Backpropagation, a fundamental algorithm for training neural networks and reducing error rates, was introduced during this time.
The rise of big data and computing power (2000s)
As data amounts and computing power increased, so did advancements in AI. Machine learning algorithms, such as deep learning, gained traction and showed remarkable performance in areas like image recognition and natural language processing.
The AI Renaissance (2010s)
The past decade witnessed significant breakthroughs in AI. Key advancements include the development of self-driving cars (albeit not yet fully realised), chatbots, virtual assistants (e.g., Siri, Alexa), and the emergence of powerful AI frameworks and libraries like TensorFlow (developed by Google) and PyTorch.
AI today
OpenAI’s GPT-3, released in 2020, is a prominent example of AI language models and sits very much at the centre of the current buzz about how AI technology will impact society. These models, including ChatGPT, use deep learning techniques to generate human-like text based on large-scale open-source training data.
People talk about ChatGPT as though it’s synonymous with AI, but it’s just a tiny piece of the puzzle. AI technology already surrounds us and is at the core of many popular tools and activities.
Netflix recommendations, social media feeds, Google searches, Spotify, Tesla’s autopilot, Grammarly, smart TVs, home security systems, intelligent lighting, personal fitness devices, Canva, Loom, YouTube, and smart cameras – to some extent, these all use AI to give us an ever-increasing degree of personalisation in our activities.
Different technologies use different types of AI, so we thought it might be helpful to explain the broad definitions of AI that you might come across:
Narrow AI
Also known as “weak AI”, narrow AI refers to AI systems that are designed to perform a specific task or a set of tasks within a limited domain. These systems excel in their specific area but lack the ability to generalise their knowledge to other domains. Examples of narrow AI include virtual personal assistants like Siri and Alexa, recommendation systems, and image recognition algorithms.
General AI
General AI, also called “strong AI” or “human-level AI”, refers to AI systems that possess the ability to understand, learn, and apply knowledge across various domains, much like a human being. These systems can potentially perform any intellectual task that a human can do.
It’s important to say that general AI remains largely hypothetical and has not been fully realised yet!
Superintelligent AI
Superintelligent AI refers to AI systems that surpass human intelligence in virtually every aspect. These hypothetical systems would possess cognitive abilities that far exceed human capacities and could potentially outperform humans in any intellectual task.
So far, this exists in the world of books and films but is something people are striving towards.
Machine Learning
Machine learning (ML) is a subset of AI that focuses on developing algorithms and models that can learn and improve from data without being explicitly programmed.
ML algorithms are trained on large datasets and can make predictions or decisions based on patterns and examples in the data. This approach is widely used in various applications, including image recognition, natural language processing, and recommendation systems.
Deep Learning
Deep learning is a subfield of machine learning that utilises artificial neural networks with multiple layers to process and learn from vast amounts of data. These neural networks are inspired by the structure and function of the human brain. Deep learning has achieved remarkable success in tasks such as image and speech recognition, natural language processing, and driving on autopilot.
Reinforcement Learning
Reinforcement learning (RL) involves training AI “agents” to make decisions in an environment to maximise rewards or minimise penalties. (An AI agent is a software program or computer system that is designed to perceive its environment, reason about it, and take actions to achieve specific objectives.)
The agent interacts with the environment, learns from feedback (rewards or punishments), and adjusts its actions accordingly. RL has been successfully used in applications like game playing, robotics, and optimisation problems, where the goal is to find the best solution from a series of options.
Expert Systems
Expert systems are AI systems that mimic the decision-making capabilities of human experts in a particular field or domain. These systems use a knowledge base and a set of rules to provide advice, recommendations, or solutions to users. Expert systems are commonly used in areas such as medical diagnosis, financial analysis, and technical support.
Natural Language Processing (NLP)
NLP focuses on enabling computers to understand, interpret, and generate human language. It involves tasks such as speech recognition, sentiment analysis, language translation, and chatbots. NLP is critical for applications like voice assistants, language translation services, and text analysis tools. It’s at the core of tools like ChatGPT or Google’s Bard, as well as automated customer service web chats, for example.
It’s probably fair to say that many of us are in equal parts excited and terrified about the potential of AI and its applications within society. Considering how it might be used for activism, for example, is a vast conversation that is evolving at speed.
For today, we want to touch on some of the clearest pros and cons of AI from the perspective of the vegan business community.
Let’s start with the potential downsides.
The downsides of AI
Automation driven by AI may lead to job displacement for certain roles. Office workers, freelancers or creatives, for example, are already facing increased competition from AI-generated content or automated platforms that perform tasks traditionally done by humans.
Look how easily people can now ask an AI art/image generator to create an image for them, for example, even specifying the style of the artist or photographer they want the piece to emulate!
The hope is that the human touch will continue to have value and offer something of an antidote to AI. Having a unique voice and perspective will be more important than ever, as will making personal connections.
The use of AI consistently raises ethical dilemmas, such as algorithmic bias, because the data comes from biased human sources and has the potential for reinforcing societal inequalities.
One example from 2016 illustrates this. The US trialled an AI algorithm designed to determine whether defendants were too dangerous to be released on bail. The algorithm looked at a wide variety of risk factors, including whether the person, their friends or family members had been arrested in the past. However, stats show that black people are at least five times more likely to be arrested than white people (in some US jurisdictions, this number rises to ten times more likely!) due to systemic bias. As a result, the algorithm unfairly predicted black defendants would break the terms of their bail (thereby recommending that it be denied), despite reoffending rates being the same for black and white defendants.
From a vegan perspective, there might be concerns about AI systems endorsing or promoting non-vegan products or ideas, not least because the datasets used by the technology are more likely to reflect the prevailing speciesist perspective.
There is the negative environmental impact of AI to consider too (we’ll cover the upsides below). All types of AI rely on significant computational resources, which can create energy consumption and electronic waste and require large data centres that put a heavy strain on local resources such as water.
The upsides of AI
Fortunately, AI does offer opportunities and possibilities too.
As a business owner, for example, having technology that can automate repetitive tasks means you will save time and effort. This may be beneficial if you’re self-employed because you’ll have more time to focus on more creative or complex work or on reaching a wider audience.
Because AI-powered systems use vast open-source datasets, they have access to a massive amount of information, which means they can tailor experiences and recommendations based on individual preferences. As vegan consumers, this should help us to connect with people, places and products that reflect our values. As vegan business owners, it should also help us to reach the people who care about the difference we want to make in the world.
Indeed, AI can help us to gather, analyse and summarise vast amounts of information quickly. This can aid vegan community members in finding scientific research, nutritional information, suppliers, solutions, and ethical considerations related to veganism.
There are environmental upsides to AI too. It’s already being used to monitor the environment, analyse climate change data, and support biodiversity conservation and restoration.
AI techniques, such as image recognition and data analysis, can help to identify and track endangered species, monitor deforestation, analyse satellite imagery, and provide vital insights for sustainable land and water management.
Of course, these pros and cons are not exhaustive! The impact of AI can and will vary depending on the context and setting.
So, there you have it – a brief whirlwind of a tour through the major milestones in the history of AI and the technologies that are likely to shape at least some aspects of the future.
In our next Ethical Globe Academy blog, we’ll take a closer look at ChatGPT and its implications for the vegan community – make sure you sign up for our newsletter so you don’t miss it! You can find the sign-up link in the footer below.